When Demis Hassabis was a young man, he helped design Theme Park, a popular computer game that gave the player a God’s-eye view of a sprawling fairground business. Ever since then, Hassabis, who leads one of the top AI labs, has been trying to attain a God’s-eye view of the world.
As CEO of DeepMind, which was founded in 2010 and acquired by Google in 2014, Hassabis has led teams of computer scientists to AI breakthroughs including solving the vexatious protein-folding problem, and beating human professionals at the complex board game Go. In April 2023, as Google’s Sundar Pichai reshuffled his company’s AI teams after the success of OpenAI’s ChatGPT, Hassabis acquired even more power. The reorganization merged DeepMind and Google’s other AI lab, Google Brain, and put Hassabis at the helm. It was an attempt by Pichai to streamline Google’s efforts toward building powerful artificial intelligence, and ward off the growing competition.
Google DeepMind, as the company’s consolidated AI lab is now known, is developing a large AI model called Gemini, which Hassabis has hinted might be able to outperform OpenAI’s GPT-4. (The model will be “multimodal,” meaning it is trained on—and can input and output—not just text but other forms of media, like images.) And much like OpenAI’s Sam Altman, Hassabis sees this as only one step in a larger pursuit of “artificial general intelligence” (AGI) that he believes could unlock scientific advances and reshape the world for the better—so long as humanity avoids the serious risks that could come from its unchecked development. (This interview has been condensed and edited for clarity.)
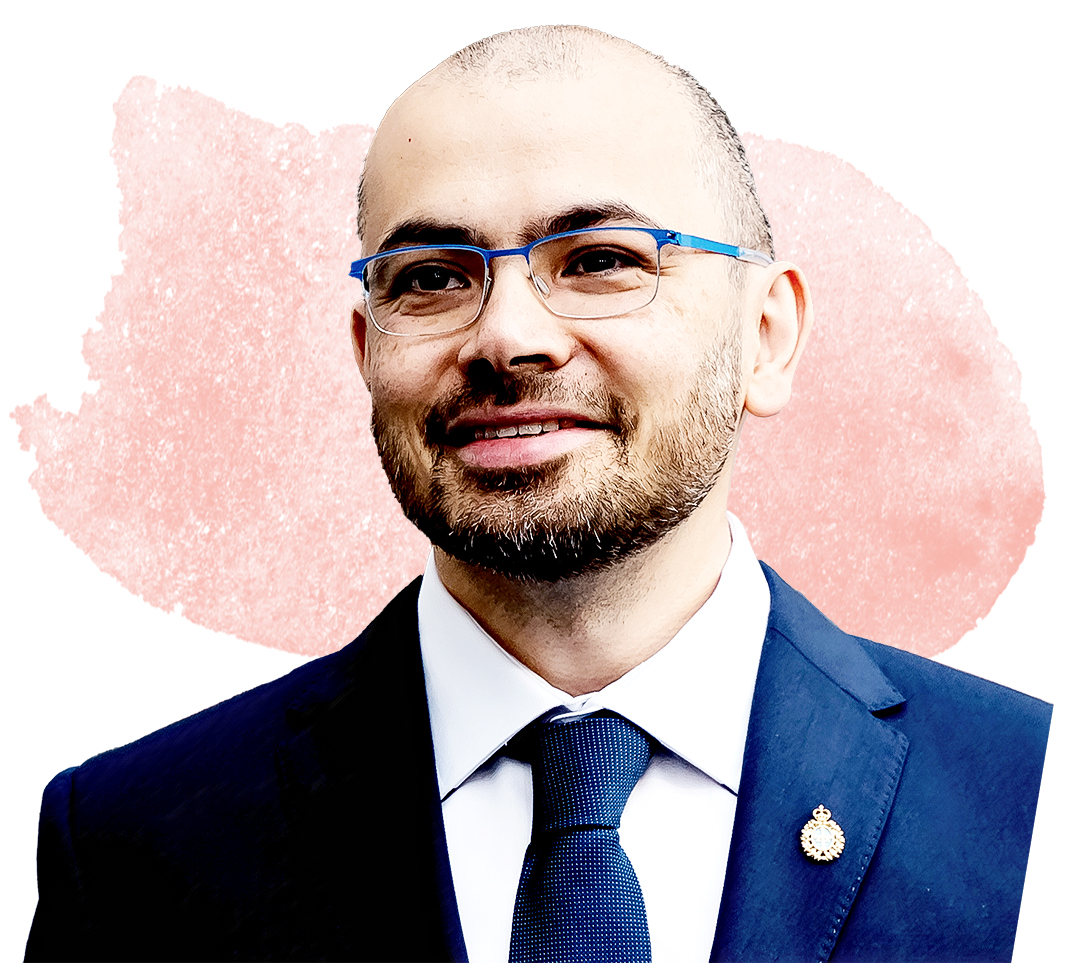
TIME: Back in April, Google announced it was combining its two separate AI labs into one, led by you. How has that changed the work you’ve been doing?
Demis Hassabis: I think it’s been great because it allows us to go faster, more streamlined, a little bit more coordinated as well, which I think is good, given how things are accelerating. In terms of the mission for the overall new unit—sometimes I like to call it a new super unit—[it’s] combining the strengths of these two amazing organizations and storied history. I want to double down on that innovation, capability, exploratory research, but also there’s a lot of coordinated big engineering that has to happen now with the large models. So that’s part of the new unit’s remit.
And then in terms of the mission, it’s a superset of what both groups were doing before. There’s obviously the advancing of the state of the art, and all the research towards AGI, and also using AI to advance science. That’s all still very much there. But also, there’s an aspect of improving billions of people’s everyday lives through AI-powered products with maybe, in the future, never-seen-before capabilities. And there’s incredible opportunity to do that at Google with the product surfaces they have. I think it’s six 2 billion-plus-user products [such as Google Search and Android], and 15 half-a-billion-user products [such as Google Drive and Photos]. So what better way to get AI out into the world and into people’s hands and to enrich their daily life? We’re very excited about continuing on all those fronts. Both groups were already doing all of that. But now it’s with even more intensity and pace, I would say.
You’re building this new model called Gemini. Should we expect it to be a bigger version of what has come before, just with more training data and more computing power, or is there something architecturally different about the way it is designed?
It’s a combination of scale, but also innovations. One of the key things about it is it will be multimodal from the ground up. We’ve done a lot of multimodal work in the past, things like Flamingo, our system to describe what’s in an image. And that has ended up underpinning a lot of multimodal work across the industry. [Gemini] is several improvements on top of that, and then it’s built in together with text models and other things. We’re also thinking about planning and memory—we’re relatively in the earliest exploratory stages of that. And you should think of Gemini as a series of models rather than a single model, although obviously, there will be individual models of different sizes. So it’s a combination of scaling and innovation, I would say. It’s very promising early results.
The large language models that we’re seeing right now have this consistent problem with so-called hallucination, or their inclination to pass off guesses as facts. It seems to be possible to make models marginally more truthful by using reinforcement-learning techniques, but it’s still unclear whether reinforcement learning and scale can solve the problem entirely. What are your thoughts right now on that question?
I think reinforcement learning can help [models] maybe even get an order of magnitude better. So quite significantly help. But to fully solve [hallucination], I think it’s going to require some other innovations. I think things like retrieval methods [and] tool use could help here. Perhaps fact-checking what [the model is] about to output, by checking with Google search as a tool, or checking in [the model’s] episodic memory banks, which maybe store facts. I think these could be good ways to get that accuracy even better. Let’s take the case that I would like to use these models for: scientific research. As you know, [chatbots’] citations often sound very plausible, but they’re made up, because they’re just plausible words. So it needs to better understand what entities are in the world. An academic paper isn’t a series of individual words; the whole citation is a unitary block, basically. So I think there are some things, like that, that a system needs to know. And therefore, it wouldn’t be appropriate to predict word by word. You need to retrieve the entire paper name, abstract, and publication venue all as one unitary piece of information.
We’re pressing on all those fronts, really. One is improving the reinforcement learning. We had some great work with Sparrow. We didn’t release Sparrow in the end as a stand-alone model, but we’ve utilized all that learning, in terms of rules adherence, and sticking to certain types of behavior. We’ve managed over time to get that improved from a 10% error rate down to 1%. So it was an order-of-magnitude improvement. The key thing is to do that without reducing the model’s lucidity, creativity, and fun-ness, in a way, and its ability to answer. So that’s the trade-off. We know how to do one or the other. It would be better if you could do both at the same time: be highly accurate, but also still be very creative and lucid.
We last spoke in November last year, before the release of ChatGPT. Even then, you issued this warning that AI is an area of research that was getting more dangerous, that we were on the cusp of these technologies becoming very disruptive to society, and that AI researchers needed to be more careful. Since then, you’ve signed your name to a letter that warned the risks of advanced AI were as serious as pandemic and nuclear war. But also since then, we’ve seen a sea change in how governments are thinking about AI policy: they’re thinking seriously about this in a way they definitely weren’t last November. Do you feel more optimistic now, or more scared, or about the same—and why?
You’re right, we spoke at an interesting moment in time, just before the latest wave of things changed everything again. I think it’s a complicated topic. I am actually quite optimistic about the situation as it is at the moment. When I talk to U.K. and U.S. government officials, they’re pretty up to speed now, which is great. That’s the plus side of the chatbot craze: that it allows the general public, politicians, and other key individuals in civil society to engage with the latest AI. I still think things like [the protein-folding breakthrough] AlphaFold are so far—perhaps I’m a little bit biased—more consequential in terms of advancing science.
Of course, language is what makes us human. So it’s clear why chatbots would resonate that much. I think the plus side is [ChatGPT] has moved the Overton window in a way so that one can discuss this, and it is a priority. What I’ve seen so far, from especially the U.K. and U.S. and a few other Western democracies, is pretty good. I think for example the U.K. white paper on AI was a very good balance between responsibility and innovation. And it’s good for it to be taken seriously.
There’s this persistent question of your independence from Google. When Google acquired DeepMind in 2014, it reportedly came with this guarantee that if DeepMind ever created AGI it would be overseen by DeepMind’s independent ethics board, rather than Google itself. But in 2021, the Wall Street Journal reported DeepMind had been involved in ultimately failed negotiations for more autonomy, including a legal structure that would prevent the powerful AI you were working on being controlled by a single corporate entity. Now, with the 2023 merger and DeepMind becoming a fully fledged part of Google, it appears from the outside that Google is tightening the leash even further and eroding whatever independence you might have had in the past. Is that what it feels like to you?
It’s actually very much the opposite. I can’t get into the past speculation, a lot of which was very inaccurate. But the ethics charter part—we’ve always had that, at original DeepMind when we were independent and when we came in [to Google], and then that developed into the [Google] AI principles. So effectively, Google has adopted that Google-wide now. We’re very comfortable with that. And there was almost no difference between that, in the end, and the DeepMind principles. That was a huge part of the input into what became the Google AI principles. So that’s all matched up. We’ve had a huge influence over [how], and I’m very happy with the way that, Google overall addresses these things. I think it’s very responsible. Our moniker is being “bold and responsible” with this technology. I think you need both. And there is a creative tension between those two words, but I think that’s intentional. We’re very comfortable with that. And now we’re all very focused on delivering the benefits of this amazing technology to the world in a thoughtful, scientific, responsible way. It doesn’t mean we’ll never make any mistakes, because it’s such a new technology. It’s moving so fast. But we want to minimize that, and maximize the benefits. So very much the opposite, really, of maybe what it looks like from the outside.
We’ve talked a lot about risks. Are there any capabilities that, if Gemini exhibited them in your testing phase, you’d decide: “No, we cannot release this”?
Yeah, I mean, it’s probably several generations down the line. I think the most pressing thing that needs to happen in the research area of AI is to come up with the right evaluation benchmarks for capabilities, because we’d all love a set of maybe even hundreds of tests, where if your system passed it, they could get a kitemark [a British certification of quality] and you say, right, this is safe to deploy in X, Y, Z way. And the government could approve that. And consumers would understand what that meant. The problem is, we don’t have those types of benchmarks currently. We have ideas about it, like, is this system capable of deception? Can it replicate itself across data centers? These are the sorts of things you might want to test for. But you need really rigorous definitions if you want to make a practical, pragmatic test for them. I think that’s the most pressing thing for the field to do as a whole. We’re all trying to do that.
The new organization we helped announce, the Frontier Model Forum, is partly about the leading companies trying to come together to do more AI safety research. Really what you want is rigorous evaluation and benchmarking technologies. And if you had those, and then a system didn’t pass that, that means you wouldn’t release it until you sorted that out. And perhaps you would do that in something like a hardened simulator, or hardened sandbox, with cybersecurity things around it. So these are the types of ideas we have, but they need to be made a little bit more concrete. I think that’s the most pressing thing to be done, in time for those types of systems when they arrive, because I think we’ve got a couple of years, probably, or more. That’s not actually a lot of time, if you think about the research that has to be done. So I’m not worried about today’s systems. But I could foresee several generations from now that we will need something more rigorous than just looking at the amount of compute they used.
- Donald Trump Is TIME's 2024 Person of the Year
- Why We Chose Trump as Person of the Year
- Is Intermittent Fasting Good or Bad for You?
- The 100 Must-Read Books of 2024
- The 20 Best Christmas TV Episodes
- Column: If Optimism Feels Ridiculous Now, Try Hope
- The Future of Climate Action Is Trade Policy
- Merle Bombardieri Is Helping People Make the Baby Decision