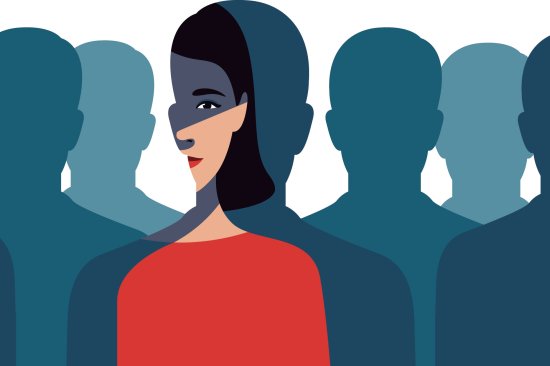
Did you hear the one about how aid workers rebuilt homes after a flood—and forgot to include kitchens? How about the entrepreneur whose product was dismissed by funders as too “niche”—but whose femtech company, Chiaro, is now on track for more than $100 million in 2020? Or the female sexual-dysfunction drug that was tested for its interaction with alcohol on 23 men … and only two women? Not finding any of these funny? Maybe that’s because they’re not jokes.
From cars that are 71% less safe for women than men (because they’ve been designed using a 50th-percentile male dummy), to voice-recognition technology that is 70% less likely to accurately understand women than men (because many algorithms are trained on 70% male data sets), to medication that doesn’t work when a woman is on her period (because women weren’t included in the clinical trials), we are living in a world that has been designed for men because for the most part, we haven’t been collecting data on women. This is the gender data gap. And if we want to design a world that works for the woman of the future as well as it works for the man of the present, we’re going to have to close it.
[time-brightcove not-tgx=”true”]
Closing this data gap is both easy and hard. It’s easy because it has a very simple solution: collect sex-disaggregated data. But it’s hard because the gender data gap is not the product of a conspiracy by a group of misogynistic data scientists. It is simply the result of an everyday bias that affects pretty much all of us: when we say human, 9 times out of 10, we mean men.
Read more of TIME’s Davos 2020 coverage
Even when we try to fix gender disparities, we still often end up using men as the default—a tendency I have christened the Henry Higgins effect, after My Fair Lady’s leading man who memorably complains, “Why can’t a woman be more like a man?” The Henry Higgins effect was visible when an executive whose voice-recognition system failed to recognize women’s voices suggested that women should undergo hours of training to fix “the many issues with women’s voices,” rather than, you know, fixing the many issues with his voice-recognition software that doesn’t recognize the voices of half the human population.
But it’s also visible in more well-meaning attempts to address gender biases. Many workplace initiatives aimed at closing gender-pay and promotion gaps focus on fixing the women, assuming that they, rather than systems that under-promote them, are the problem. Women need confidence training. They need to be taught to negotiate for pay raises. Well, actually, the evidence suggests that women are asking for pay raises as often as men—they’re just less likely to get them. Perhaps the issue here is not the women, but a system that doesn’t account for gender bias?
There are reasons beyond fairness to fix systems that are arguably primed to over-promote men: homogeneity is bad for business. Even with the best will in the world, a group of white middle-class men from America are going to have gaps in their knowledge, and they aren’t necessarily going to know what those gaps are. Which is how you end up with a “comprehensive” health tracker app that can’t track your period. And I don’t believe Apple hates periods; I believe that Apple forgot periods exist.
The gender data gap and its default male origins have been disadvantaging women for millennia, but in a world where we increasingly outsource our decision-making to algorithms trained on data with a great big hole in it, this problem is set to get a lot more serious very quickly. And if we don’t choose to correct the mistakes of the past now, we will blunder into a future where we have literally coded them in.
Part of the problem is our blind faith in AI. When tech entrepreneur David Heinemeier Hansson complained to Apple that his wife was given a limit for her credit card 20 times lower than his, despite having a higher credit score, he was informed by workers at the company that it was not discrimination, it was “just the algorithm.” Having accepted that we humans are hopelessly flawed and biased, we are turning to artificial intelligence to save us.
But algorithms are only as good as the data we feed them, and when it comes to women, that data is practically non-existent. Worse, algorithms amplify our biases back to us. One University of Washington study found that when an algorithm was trained on an image data set where pictures of cooking were 33% more likely to feature women than men, the algorithm increased the disparity to 68%. That is, pictures of men were labeled as female just because they were in front of a stove.
Labeling a man as female may not feel like an egregious example of algorithmic bias, but imagine an amplified bias like that let loose in hiring. This has already happened to Amazon, which had to abandon an AI program after it favored men over women for suggested hiring. And that’s just one algorithm that we know about: 72% of CVs in the U.S. never reached human eyes as of 2016, and robots trained on the posture, facial expressions and vocal tone of “top-performing employees” have been introduced to interview processes. Are these top-performing employees gender and ethnically diverse, and if not, has the algorithm accounted for this? We often don’t know because most algorithms are protected as proprietary software, but the evidence isn’t promising.
Even more concerning is the introduction of AI into medical diagnostics, where the data gap and a male-biased curriculum already leaves women 50% more likely to be misdiagnosed if they have a heart attack. And yet there is little evidence of developers’ accounting for this bias. A recent paper detailed an algorithm that was intended to predict heart attacks five years before they happen: it was trained on heavily male-dominated studies, even though we know there are major sex differences in risk factors for cardiovascular disease such as diabetes and smoking. So will this AI predict heart attacks in women? It’s impossible to say, because the paper doesn’t include enough sex-disaggregated data.
There are solutions to these problems if we choose to acknowledge them. A 2016 paper on “word-embeddings” (learning techniques that are essential for search algorithms) explained a new methodology that reduced gender stereotyping (e.g., “He is to doctor as she is to nurse”) by over two-thirds, while leaving gender-appropriate word associations (e.g., “He is to prostate cancer as she is to ovarian cancer”) intact. The authors of the University of Washington image-labeling study devised a new algorithm that decreased bias amplification by 47.5%. But these examples are very much the exception.
If we want to design a just future, we must acknowledge—and mitigate against—this fundamental bias that frames women as atypical. Women are not a confounding factor to be eliminated from research like so many rogue data points. In this new world where data is king (and I use that term advisedly), it’s time for us to finally start counting women as the entirely average humans that they are.
Criado Perez is the author of Invisible Women: Data Bias in a World Designed for Men
TIME’s Davos 2020 issue was produced in partnership with the World Economic Forum.