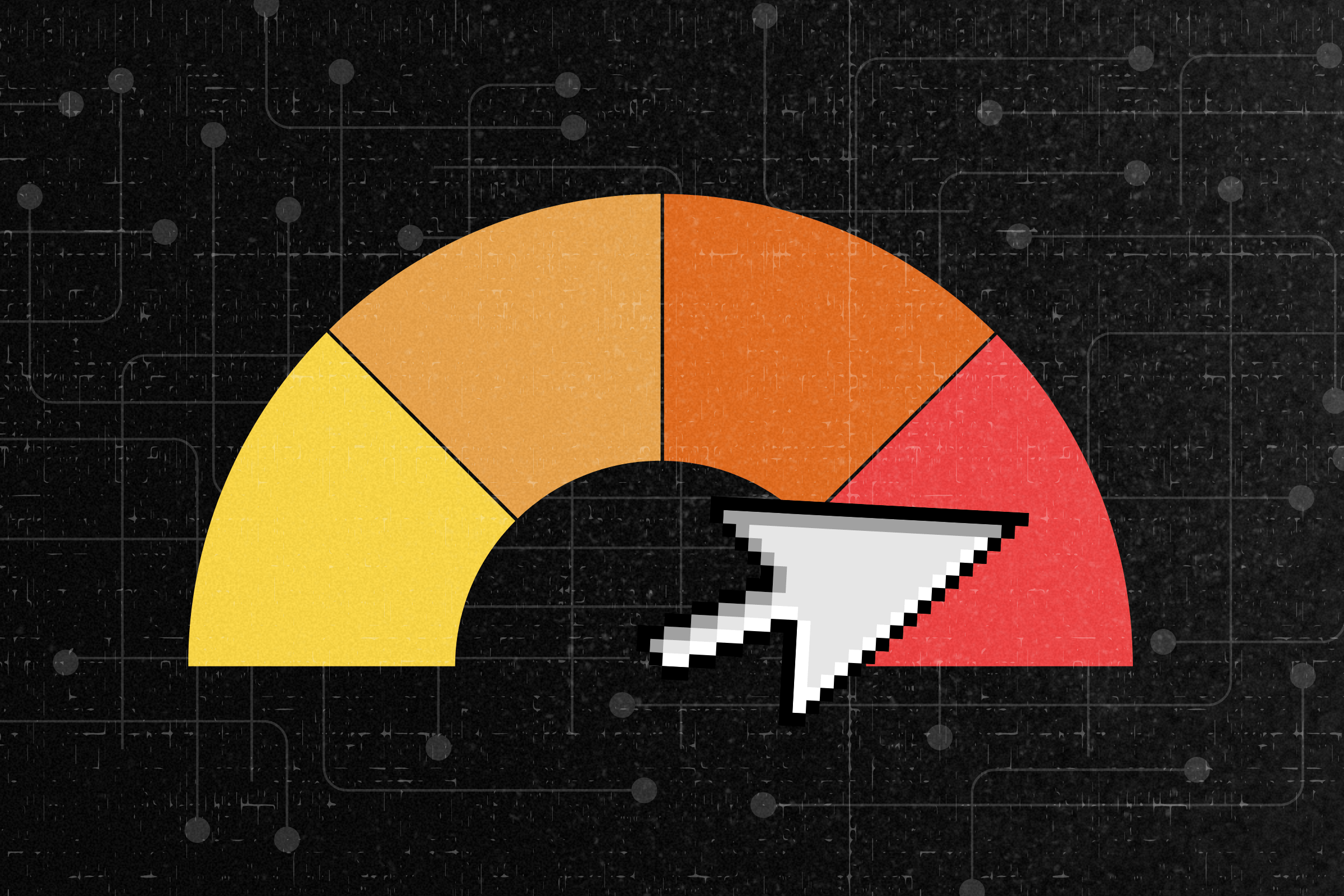
Beth Barnes and three of her colleagues sit cross-legged in a semicircle on a damp lawn on the campus of the University of California, Berkeley. They are describing their attempts to interrogate artificial intelligence chatbots.
“They are, in some sense, these vast alien intelligences,” says Barnes, 26, who is the founder and CEO of Model Evaluation and Threat Research (METR), an AI-safety nonprofit. “They know so much about whether the next word is going to be ‘is’ versus ‘was.’ We're just playing with a tiny bit on the surface, and there's all this, miles and miles underneath,” she says, gesturing at the potentially immense depths of large language models’ capabilities. (Large language models, such as OpenAI’s GPT-4 and Anthropic’s Claude, are giant AI systems that are trained by predicting the next word for a vast amount of text, and that can answer questions and carry out basic reasoning and planning.)
Researchers at METR look a lot like Berkeley students—the four on the lawn are in their twenties and dressed in jeans or sweatpants. But rather than attending lectures or pulling all-nighters in the library, they spend their time probing the latest and most powerful AI systems to try and determine whether, if you asked just right, they could do something dangerous. As they explain how they try to ascertain whether the current generation of chatbots or the next could cause a catastrophe, they pick at the grass. They may be young, but few people have thought about how to elicit danger from AIs as much as they have.
Two of the world’s most prominent AI companies—OpenAI and Anthropic—have worked with METR as part of their efforts to safety-test their AI models. The U.K. government partnered with METR as part of its efforts to start safety-testing AI systems, and President Barack Obama called METR out as a civil society organization working to meet the challenges posed by AI in his statement on President Joe Biden’s AI Executive Order.
“It does feel like we're trying to understand the experience of being a language model sometimes,” says Haoxing Du, a METR researcher, describing the act of putting oneself in a chatbot’s shoes, an endeavor she and her colleagues wryly refer to as model psychology.
As warnings about the dangers that powerful future AI systems could pose have grown louder, lawmakers and executives have begun to converge on an ostensibly straightforward plan: test the AI models to see if they are indeed dangerous. But Barnes, along with many AI-safety researchers, says that this plan might be betting the house on safety tests that don’t yet exist.
How to test an AI
In the summer of 2022, Barnes decided to leave OpenAI, where she had spent three years as a researcher working on a range of safety and forecasting projects. This was, in part, a pragmatic decision—she felt that there should be some neutral third-party organization that was developing AI evaluations. But Barnes also says that she was one of the most openly critical OpenAI employees, and that she felt she would be more comfortable and more effective advocating for safety practices from the outside. “I think I am a very open and honest person,” she says. “I am not very good at navigating political things and not making disagreements pretty obvious.”
Read More: Employees at Top AI Labs Fear Safety Is an Afterthought, Report Says
She founded METR solo that year. It was originally called ARC Evals, under the umbrella of the AI-safety organization Alignment Research Center (ARC), but spun out in December 2023 to become METR. It now has 20 employees, including Barnes.
While METR is the only safety-testing organization to have partnered with leading AI companies, there are researchers across governments, nonprofits, and in industry working on evaluations that test for various potential dangers, such as whether an AI model could assist in carrying out a cyberattack or releasing a bioweapon. METR’s initial focus was assessing whether an AI model could self-replicate, using its smarts to earn money and acquire more computational resources, and using those resources to make more copies of itself, ultimately spreading across the internet. Its focus has since broadened to assessing whether AI models can act autonomously, by navigating the internet and carrying out complex tasks without oversight.
METR focuses on testing for this because it requires less specialized expertise than, say, biosecurity testing, and because METR is particularly concerned about the damage an AI system could do if it could act fully independently and therefore could not simply be turned off, says Barnes.
The threat that METR first focused on is on the minds of government officials, too. Voluntary commitments secured by the Biden Administration from 15 leading AI companies include a responsibility to test new models for the capacity to “make copies of themselves or ‘self-replicate.’”
Currently, if one were to ask a state-of-the-art AI, such as Google DeepMind’s Gemini or OpenAI’s GPT-4, how it would go about spreading copies of itself around the internet, its response would be vague and lackluster, even if the safety protections that typically prevent AI systems from responding to problematic prompts were stripped away. Barnes and her team believe that nothing on the market today is capable of self-replication, but they don’t think this will last. “It seems pretty hard to be confident that it's not gonna happen within five years,” says Barnes.
METR wants to be able to detect whether an AI is starting to pick up the ability to self-replicate and act autonomously long before it can truly do so. To achieve this, researchers try to give the models as many advantages as possible. This includes trying to find the prompts that produce the best-possible performance, giving the AI tools that would help in the task of self-replicating, and giving it further training on tasks that it would need to accomplish in order to self-replicate, such as searching through a large number of files for relevant information. Even with all of the advantages METR can confer, current AI models are reassuringly bad at this.
If an AI armed with all of these advantages still gets nowhere near self-replication and autonomous action based on METR’s tests, METR is relatively confident the model won’t be able to fend for itself once released into the world—and that it wouldn’t even if it were made slightly more powerful. However, as models become increasingly capable, METR is likely to become less sure of its assessments, Barnes says.
Evaluation enthusiasm
Speaking at the White House before he signed his administration’s AI executive order in October, President Biden said that companies must “tell the government about the large-scale AI systems they’re developing and share rigorous independent test results to prove they pose no national security or safety risk to the American people.” Biden’s executive order tasked the National Institute of Standards and Technology (NIST) with establishing guidelines for testing AI systems to make sure they are safe. Once the guidelines have been written, companies will need to report the results of their tests to the government. Similarly, the E.U. AI Act requires companies that create particularly powerful AI systems to safety-test them.
The Bletchley Declaration, signed by 29 countries including the U.S. and China at the U.K. AI Safety Summit in November, says that actors developing the most powerful AI systems have a responsibility to ensure their systems are safe “through systems for safety-testing, through evaluations, and by other appropriate measures.”
It’s not just governments that are enthused about the idea of safety-testing. Both OpenAI and Anthropic have published detailed plans for future AI development, which involve verifying their systems are safe before deploying them or building more powerful systems.
Safety tests, then, are set to play a pivotal role in the strategies for safe AI development of both companies and governments. But no one involved in developing these evaluations claims they’re airtight. “The evals are not ready,” says Chris Painter, METR’s policy director. “There's a real and material execution question about whether the tests will be ready with the fidelity that would be needed in the next year. And AI progress is going to keep going in the next year.”
Government officials express similar sentiments. “I'm not going to pretend to say that we—NIST—have all of the answers,” says Elham Tabassi, chief technology officer at the U.S. AI Safety Institute. “Coming up with a systematic way of evaluating is exactly what you're after… we as a community quite don't have the answer for that.”
Read More: Researchers Develop New Technique to Wipe Dangerous Knowledge From AI Systems
And even inside the labs, researchers are aware of the tests’ shortcomings. “We're in early stages, where we have promising signals that we're excited about,” says Tejal Patwardhan, a member of technical staff in the team at OpenAI that develops safety tests—referred to as the Preparedness team. “But I wouldn't say we're 1,000% sure about everything.”
The problem with safety-testing
Given that large language models are a very new technology, it makes sense that no one yet knows how to safety-test them. But at the same time, AI is progressing rapidly, and many people developing the most powerful systems believe that their creations might outsmart humans this decade.
For those concerned about risks from powerful AI systems, this is an alarming state of affairs. “We have no idea how to actually understand and evaluate our models,” says Connor Leahy, CEO of AI safety company Conjecture, who recently told TIME that humanity might have less than five years before AI could pose an existential threat and advocates for an international agreement banning the development of AI models above a certain size.
METR and others could be complicit in “safetywashing” by justifying continued dangerous AI development based on tests that are still a long way from guaranteeing safety, warns Leahy. “You shouldn't build policy on this. It's in the interest of the corporations and the lobbyists to take these extremely scientifically early results and then puff them up into this huge thing.”
Barnes, who also worries about risks from powerful AI systems, agrees that the best solution would be to stop building ever-larger AI models until the potential risks are better understood and managed. But she argues that METR’s efforts are a pragmatic step that improves things in the absence of such a moratorium, and that it’s better for companies to publish a flawed safety-testing plan that can be improved upon than not publish one at all. While OpenAI and Anthropic have published such plans and Google DeepMind CEO Demis Hassabis recently said that his company would do the same soon, companies such as Meta, Cohere, and Mistral are yet to do the same, Barnes notes. Meta and Cohere’s leadership argue that the sorts of risks that METR and others test for are farfetched.
Aside from the issue of whether the tests work, there’s the question of whether METR is in a position to administer them, says Leahy, noting that Barnes previously worked at OpenAI and that companies are currently under no obligation to grant METR, or any other organization, the access required to safety-test their models, meaning evaluators risk losing access if they are critical.
METR has taken a number of practical steps to increase its independence, such as requiring staff to sell any financial interests in companies developing the types of system that they test, says Barnes. But ultimately, METR is trying to walk the line between putting pressure on labs and retaining the right to test their models, and it would be better if the government required developers to grant access to organizations like METR, she says. At least for now, it makes more sense to think of METR’s work with AI companies as a research collaboration than a mechanism for external oversight, says Painter.
Read More: The 3 Most Important AI Policy Milestones of 2023
More from TIME
Voluntary safety-testing, whether carried out by METR or the AI companies, cannot be relied upon, says Dan Hendrycks, executive director of nonprofit the Center for AI Safety and the safety advisor to Elon Musk’s AI company xAI. More fundamentally, the focus on testing has distracted from “real governance things,” he argues, such as passing laws that would ensure AI companies are liable for damages caused by their models and promoting international cooperation.
Here, Barnes essentially agrees: “I definitely don't think that the only AI safety work should be evaluations,” she says. But even with the spotlight on safety-testing, there’s still a lot of work to be done, she says.
“By the time that we have models that are just really risky, there are a lot of things that we have to have in place,” she says. “We're just pretty far off now.”
More Must-Reads from TIME
- Why Trump’s Message Worked on Latino Men
- What Trump’s Win Could Mean for Housing
- The 100 Must-Read Books of 2024
- Sleep Doctors Share the 1 Tip That’s Changed Their Lives
- Column: Let’s Bring Back Romance
- What It’s Like to Have Long COVID As a Kid
- FX’s Say Nothing Is the Must-Watch Political Thriller of 2024
- Merle Bombardieri Is Helping People Make the Baby Decision
Write to Will Henshall at will.henshall@time.com