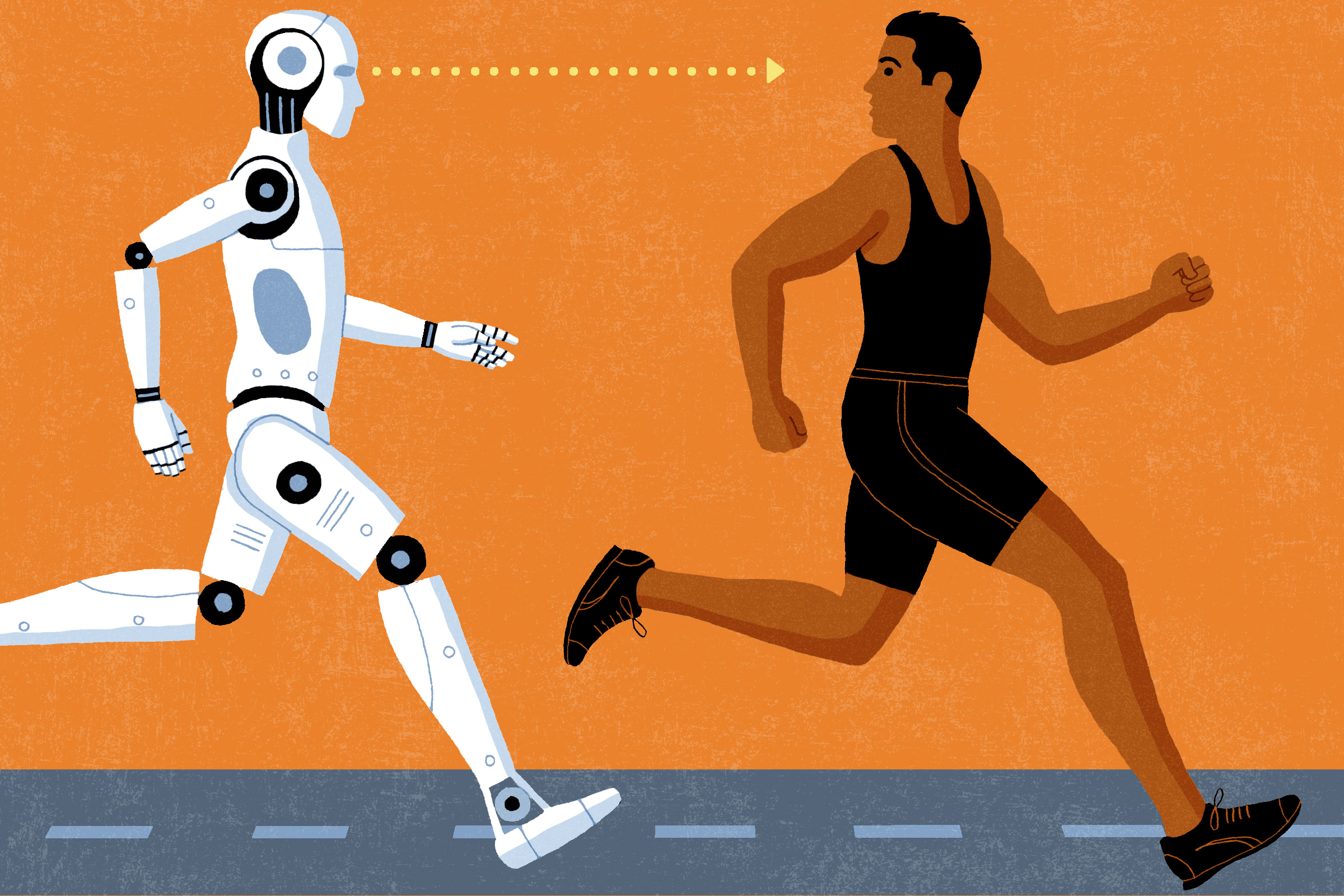
In 1960, Herbert Simon, who went on to win both the Nobel Prize for economics and the Turing Award for computer science, wrote in his book The New Science of Management Decision that “machines will be capable, within 20 years, of doing any work that a man can do.”
History is filled with exuberant technological predictions that have failed to materialize. Within the field of artificial intelligence, the brashest predictions have concerned the arrival of systems that can perform any task a human can, often referred to as artificial general intelligence, or AGI.
So when Shane Legg, Google DeepMind’s co-founder and chief AGI scientist, estimates that there’s a 50% chance that AGI will be developed by 2028, it might be tempting to write him off as another AI pioneer who hasn’t learnt the lessons of history.
Still, AI is certainly progressing rapidly. GPT-3.5, the language model that powers OpenAI’s ChatGPT was developed in 2022, and scored 213 out of 400 on the Uniform Bar Exam, the standardized test that prospective lawyers must pass, putting it in the bottom 10% of human test-takers. GPT-4, developed just months later, scored 298, putting it in the top 10%. Many experts expect this progress to continue.
Read More: 4 Charts That Show Why AI Progress Is Unlikely to Slow Down
Legg’s views are common among the leadership of the companies currently building the most powerful AI systems. In August, Dario Amodei, co-founder and CEO of Anthropic, said he expects a “human-level” AI could be developed in two to three years. Sam Altman, CEO of OpenAI, believes AGI could be reached sometime in the next four or five years.
But in a recent survey the majority of 1,712 AI experts who responded to the question of when they thought AI would be able to accomplish every task better and more cheaply than human workers were less bullish. A separate survey of elite forecasters with exceptional track records shows they are less bullish still.
The stakes for divining who is correct are high. Legg, like many other AI pioneers, has warned that powerful future AI systems could cause human extinction. And even for those less concerned by Terminator scenarios, some warn that an AI system that could replace humans at any task might replace human labor entirely.
The scaling hypothesis
Many of those working at the companies building the biggest and most powerful AI models believe that the arrival of AGI is imminent. They subscribe to a theory known as the scaling hypothesis: the idea that even if a few incremental technical advances are required along the way, continuing to train AI models using ever greater amounts of computational power and data will inevitably lead to AGI.
There is some evidence to back this theory up. Researchers have observed very neat and predictable relationships between how much computational power, also known as “compute,” is used to train an AI model and how well it performs a given task. In the case of large language models (LLM)—the AI systems that power chatbots like ChatGPT—scaling laws predict how well a model can predict a missing word in a sentence. OpenAI CEO Sam Altman recently told TIME that he realized in 2019 that AGI might be coming much sooner than most people think, after OpenAI researchers discovered the scaling laws.
Read More: 2023 CEO of the Year: Sam Altman
Even before the scaling laws were observed, researchers have long understood that training an AI system using more compute makes it more capable. The amount of compute being used to train AI models has increased relatively predictably for the last 70 years as costs have fallen.
Early predictions based on the expected growth in compute were used by experts to anticipate when AI might match (and then possibly surpass) humans. In 1997, computer scientist Hans Moravec argued that cheaply available hardware will match the human brain in terms of computing power in the 2020s. An Nvidia A100 semiconductor chip, widely used for AI training, costs around $10,000 and can perform roughly 20 trillion FLOPS, and chips developed later this decade will have higher performance still. However, estimates for the amount of compute used by the human brain vary widely from around one trillion floating point operations per second (FLOPS) to more than one quintillion FLOPS, making it hard to evaluate Moravec’s prediction. Additionally, training modern AI systems requires a great deal more compute than running them, a fact that Moravec’s prediction did not account for.
More recently, researchers at nonprofit Epoch have made a more sophisticated compute-based model. Instead of estimating when AI models will be trained with amounts of compute similar to the human brain, the Epoch approach makes direct use of scaling laws and makes a simplifying assumption: If an AI model trained with a given amount of compute can faithfully reproduce a given portion of text—based on whether the scaling laws predict such a model can repeatedly predict the next word almost flawlessly—then it can do the work of producing that text. For example, an AI system that can perfectly reproduce a book can substitute for authors, and an AI system that can reproduce scientific papers without fault can substitute for scientists.
Some would argue that just because AI systems can produce human-like outputs, that doesn’t necessarily mean they will think like a human. After all, Russell Crowe plays Nobel Prize-winning mathematician John Nash in the 2001 film, A Beautiful Mind, but nobody would claim that the better his acting performance, the more impressive his mathematical skills must be. Researchers at Epoch argue that this analogy rests on a flawed understanding of how language models work. As they scale up, LLMs acquire the ability to reason like humans, rather than just superficially emulating human behavior. However, some researchers argue it's unclear whether current AI models are in fact reasoning.
Epoch’s approach is one way to quantitatively model the scaling hypothesis, says Tamay Besiroglu, Epoch’s associate director, who notes that researchers at Epoch tend to think AI will progress less rapidly than the model suggests. The model estimates a 10% chance of transformative AI—defined as “AI that if deployed widely, would precipitate a change comparable to the industrial revolution”—being developed by 2025, and a 50% chance of it being developed by 2033. The difference between the model’s forecast and those of people like Legg is probably largely down to transformative AI being harder to achieve than AGI, says Besiroglu.
Asking the experts
Although many in leadership positions at the most prominent AI companies believe that the current path of AI progress will soon produce AGI, they’re outliers. In an effort to more systematically assess what the experts believe about the future of artificial intelligence, AI Impacts, an AI safety project at the nonprofit Machine Intelligence Research Institute, surveyed 2,778 experts in fall 2023, all of whom had published peer-reviewed research in prestigious AI journals and conferences in the last year.
Among other things, the experts were asked when they thought “high-level machine intelligence,” defined as machines that could “accomplish every task better and more cheaply than human workers” without help, would be feasible. Although the individual predictions varied greatly, the average of the predictions suggests a 50% chance that this would happen by 2047, and a 10% chance by 2027.
Like many people, the experts seemed to have been surprised by the rapid AI progress of the last year and have updated their forecasts accordingly—when AI Impacts ran the same survey in 2022, researchers estimated a 50% chance of high-level machine intelligence arriving by 2060, and a 10% chance by 2029.
The researchers were also asked when they thought various individual tasks could be carried out by machines. They estimated a 50% chance that AI could compose a Top 40 hit by 2028 and write a book that would make the New York Times bestseller list by 2029.
The superforecasters are skeptical
Nonetheless, there is plenty of evidence to suggest that experts don’t make good forecasters. Between 1984 and 2003, social scientist Philip Tetlock collected 82,361 forecasts from 284 experts, asking them questions such as: Will Soviet leader Mikhail Gorbachev be ousted in a coup? Will Canada survive as a political union? Tetlock found that the experts’ predictions were often no better than chance, and that the more famous an expert was, the less accurate their predictions tended to be.
Next, Tetlock and his collaborators set out to determine whether anyone could make accurate predictions. In a forecasting competition launched by the U.S. Intelligence Advanced Research Projects Activity in 2010, Tetlock’s team, the Good Judgement Project (GJP), dominated the others, producing forecasts that were reportedly 30% more accurate than intelligence analysts who had access to classified information. As part of the competition, the GJP identified “superforecasters”—individuals who consistently made above-average accuracy forecasts. However, although superforecasters have been shown to be reasonably accurate for predictions with a time horizon of two years or less, it's unclear whether they’re also similarly accurate for longer-term questions such as when AGI might be developed, says Ezra Karger, an economist at the Federal Reserve Bank of Chicago and research director at Tetlock’s Forecasting Research Institute.
When do the superforecasters think AGI will arrive? As part of a forecasting tournament run between June and October 2022 by the Forecasting Research Institute, 31 superforecasters were asked when they thought Nick Bostrom—the controversial philosopher and author of the seminal AI existential risk treatise Superintelligence—would affirm the existence of AGI. The median superforecaster thought there was a 1% chance that this would happen by 2030, a 21% chance by 2050, and a 75% chance by 2100.
Who’s right?
All three approaches to predicting when AGI might be developed—Epoch’s model of the scaling hypothesis, and the expert and superforecaster surveys—have one thing in common: there’s a lot of uncertainty. In particular, the experts are spread widely, with 10% thinking it's as likely as not that AGI is developed by 2030, and 18% thinking AGI won’t be reached until after 2100.
Still, on average, the different approaches give different answers. Epoch’s model estimates a 50% chance that transformative AI arrives by 2033, the median expert estimates a 50% probability of AGI before 2048, and the superforecasters are much further out at 2070.
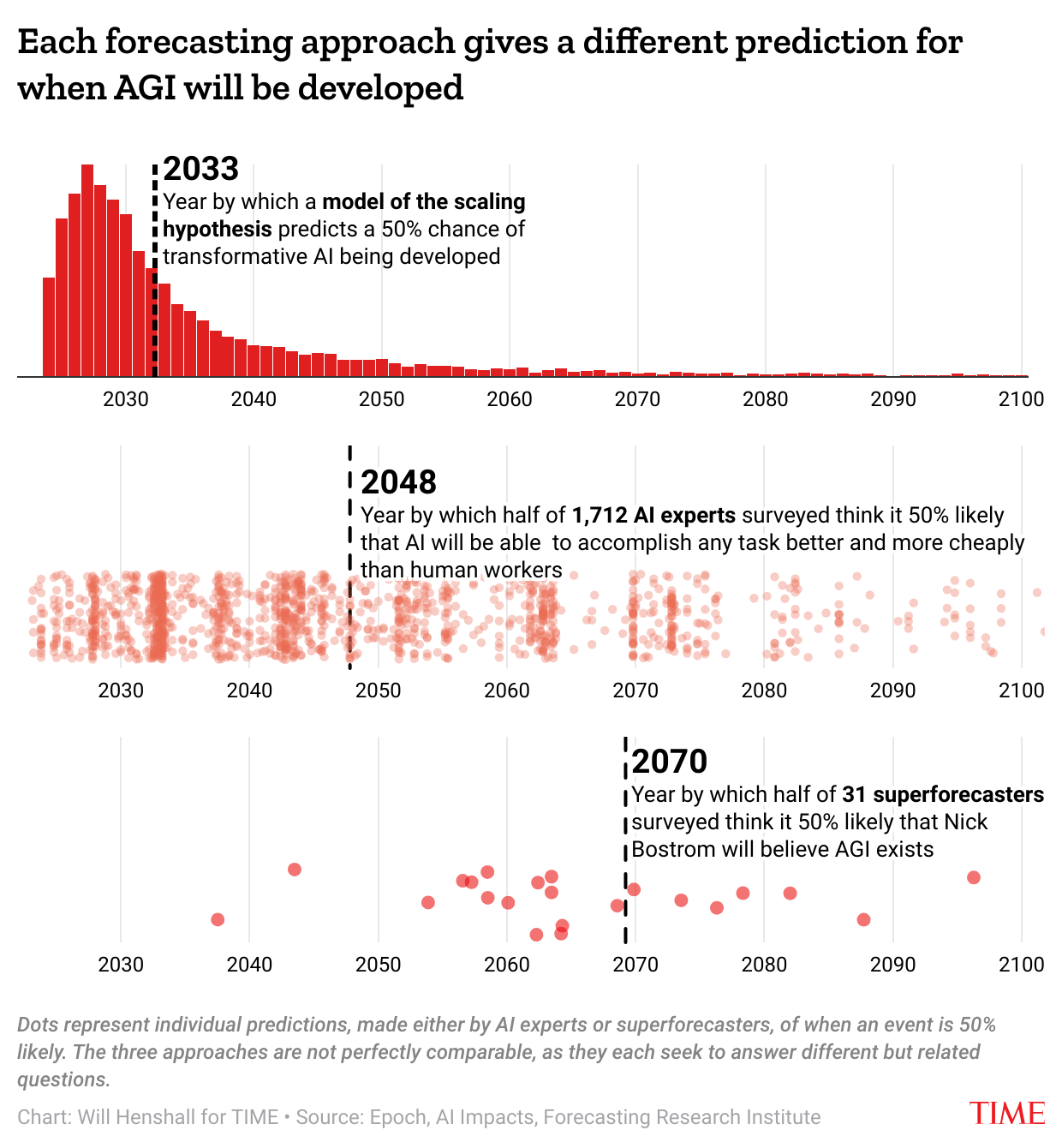
There are many points of disagreement that feed into debates over when AGI might be developed, says Katja Grace, who organized the expert survey as lead researcher at AI Impacts. First, will the current methods for building AI systems, bolstered by more compute and fed more data, with a few algorithmic tweaks, be sufficient? The answer to this question in part depends on how impressive you think recently developed AI systems are. Is GPT-4, in the words of researchers at Microsoft, the sparks of AGI? Or is this, in the words of philosopher Hubert Dreyfus, “like claiming that the first monkey that climbed a tree was making progress towards landing on the moon?”
Second, even if current methods are enough to achieve the goal of developing AGI, it's unclear how far away the finish line is, says Grace. It’s also possible that something could obstruct progress on the way, for example a shortfall of training data.
Finally, looming in the background of these more technical debates are people’s more fundamental beliefs about how much and how quickly the world is likely to change, Grace says. Those working in AI are often steeped in technology and open to the idea that their creations could alter the world dramatically, whereas most people dismiss this as unrealistic.
The stakes of resolving this disagreement are high. In addition to asking experts how quickly they thought AI would reach certain milestones, AI Impacts asked them about the technology’s societal implications. Of the 1,345 respondents who answered questions about AI’s impact on society, 89% said they are substantially or extremely concerned about AI-generated deepfakes and 73% were similarly concerned that AI could empower dangerous groups, for example by enabling them to engineer viruses. The median respondent thought it was 5% likely that AGI leads to “extremely bad,” outcomes, such as human extinction.
Given these concerns, and the fact that 10% of the experts surveyed believe that AI might be able to do any task a human can by 2030, Grace argues that policymakers and companies should prepare now.
Preparations could include investment in safety research, mandatory safety testing, and coordination between companies and countries developing powerful AI systems, says Grace. Many of these measures were also recommended in a paper published by AI experts last year.
“If governments act now, with determination, there is a chance that we will learn how to make AI systems safe before we learn how to make them so powerful that they become uncontrollable,” Stuart Russell, professor of computer science at the University of California, Berkeley, and one of the paper’s authors, told TIME in October.
More Must-Reads From TIME
- The 100 Most Influential People of 2024
- Coco Gauff Is Playing for Herself Now
- Scenes From Pro-Palestinian Encampments Across U.S. Universities
- 6 Compliments That Land Every Time
- If You're Dating Right Now , You're Brave: Column
- The AI That Could Heal a Divided Internet
- Fallout Is a Brilliant Model for the Future of Video Game Adaptations
- Want Weekly Recs on What to Watch, Read, and More? Sign Up for Worth Your Time
Write to Will Henshall at will.henshall@time.com