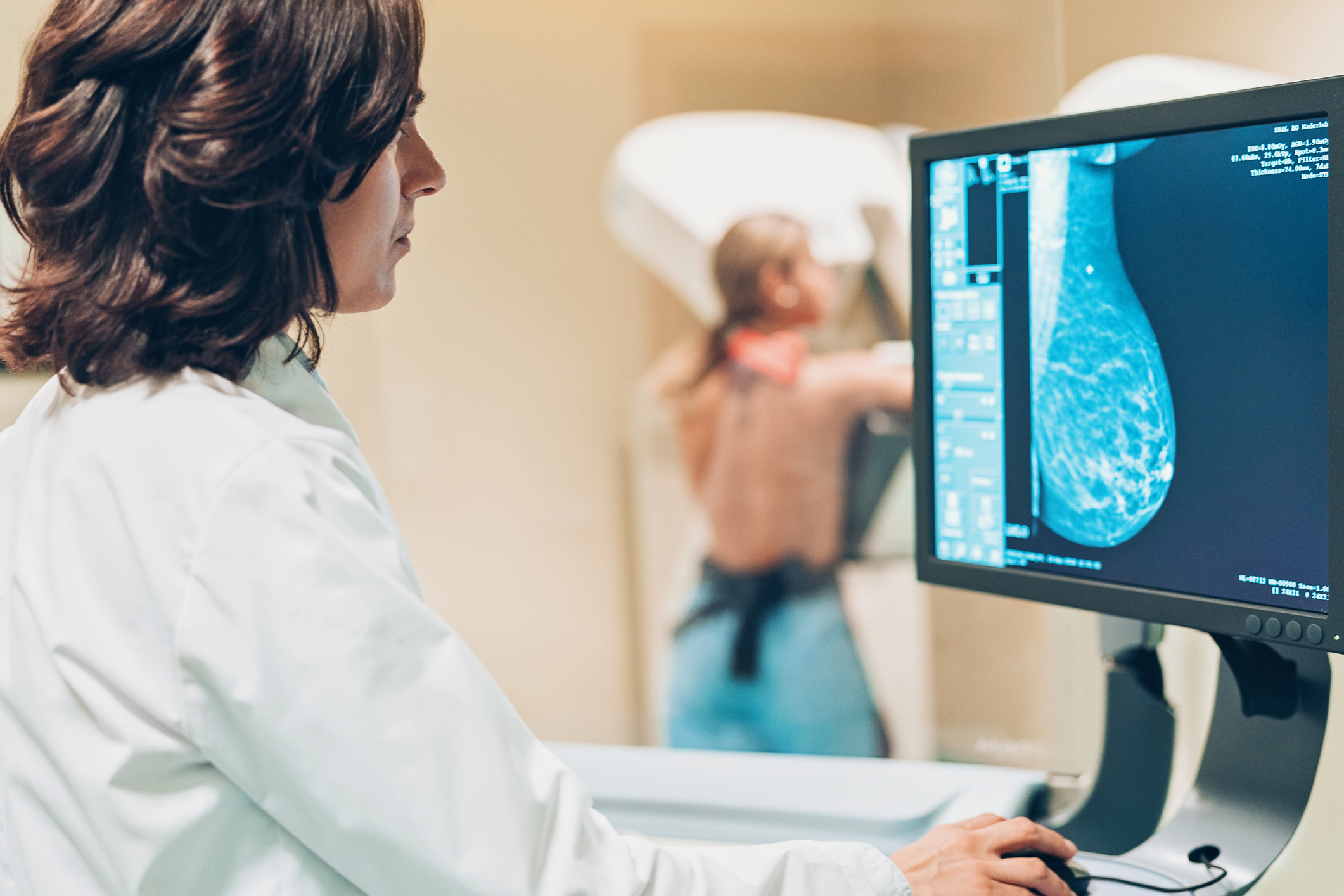
While there has been controversy over when and how often women should be screened for breast cancer using mammograms, studies consistently show that screening can lead to earlier detection of the disease, when it’s more treatable. So improving how effectively mammograms can detect abnormal growths that could be cancerous is a priority in the field.
AI could play a role in accomplishing that—computer-based machine learning might help doctors to read mammograms more accurately. In a study published Jan. 1 in Nature, researchers from Google Health, and from universities in the U.S. and U.K., report on an AI model that reads mammograms with fewer false positives and false negatives than human experts. The algorithm, based on mammograms taken from more than 76,000 women in the U.K. and more than 15,000 in the U.S., reduced false positive rates by nearly 6% in the U.S., where women are screened every one to two years, and by 1.2% in the U.K., where women are screened every three years. The AI model also lowered false negatives by more than 9% in the U.S. and by nearly 3% in the U.K.
“Reading mammograms is the perfect problem for machine learning and AI,” says Dr. Mozziyar Etemadi, research assistant professor of anesthesiology and biomedical engineering at Northwestern University and one of the paper’s co-authors. “AI excels when it has to do the same task over and over again, and has to find the one thing that might appear one time out of 10,000. But I honestly did not expect it to work this much better [than doctors]. I was surprised.”
It’s an improvement over other, smaller studies on AI and mammograms; in one notable one, a machine performed as well as 101 radiologists in reading scans, meaning both had similar rates of false positives and false negatives. This recent study is one of the most statistically significant to date due to its large dataset, and because of the fact that the AI model didn’t just match, but outperformed doctors.
Once the team knew the AI could be trained to effectively read mammograms on both the UK and U.S. data set, they ran another test: if they trained the algorithm on the U.K. data, then used it on the U.S. data, could it still accurately predict breast cancer? Indeed, the AI had 3.5% less false positives and 8.1% less false negatives than the doctors. “That’s encouraging because in the real-world situations where we want to get our models, that’s what is going to happen; it will be used on populations on which it hasn’t necessarily been trained,” says Shravya Shetty, technical lead at Google Health.
The algorithm was based on one the Google Research team initially developed for detecting diabetic retinopathy from retinal scans, then reconfigured to read CT scans for lung cancer. One advantage of the Google platform, says Etemadi, is its unmatched computing power. As the resolution of mammography images has improved in recent years, they’ve become so dense with data that the human eye (even one belonging to a highly trained radiologist) may not be able to process completely. Most hospital computer systems aren’t powerful enough to even load the entirety of information provided in modern mammograms, so radiologists see only selected information. Google’s computing power allowed the algorithm to process nearly every available pixel.
In order for an AI algorithm to recognize abnormal lesions in breast tissue, the model needs to be trained with a huge number of mammogram images—the more the better—and those images need to be linked to outcomes such as cancer, or benign lesions. The size of the database in this study provides more confidence that the algorithm could potentially be useful in a medical setting.
For now, experts see AI as a supplement to radiologists reading mammogram images, rather than as a replacement. For example, AI models could perform the first pass of evaluations, leaving experts, who fold in other valuable information such as a woman’s family history of cancer, her health status, and more, to interpret more difficult cases. “Health care is being squeezed with the number of patients increasing and the amount of time that doctors have to see patients decreasing. So tools like these are what every physician is hoping for,” says Etemadi. “We just have to better understand when tools like AI help and when it doesn’t and ultimately come up with the combination of technology and human contributions what will ultimately improve care and make it more efficient.”
More Must-Reads from TIME
- Breaking Down the 2024 Election Calendar
- How Nayib Bukele’s ‘Iron Fist’ Has Transformed El Salvador
- What if Ultra-Processed Foods Aren’t as Bad as You Think?
- How Ukraine Beat Russia in the Battle of the Black Sea
- Long COVID Looks Different in Kids
- How Project 2025 Would Jeopardize Americans’ Health
- What a $129 Frying Pan Says About America’s Eating Habits
- The 32 Most Anticipated Books of Fall 2024
Contact us at letters@time.com