The following feature is excerpted from TIME Artificial Intelligence: The Future of Humankind, available at retailers and at the Time Shop and Amazon.
Medicine is both art and science. While any doctor will quickly credit her rigorous medical training in the nuts and bolts of how the human body works, she will just as adamantly school you on how virtually all of the decisions she makes—about how to diagnose disease and how best to treat it—are equally the product of some less tangible measures: her experience from previous patients; her cumulative years of watching and learning from patients, colleagues and the human body.
Which is why the idea of introducing machines into medicine seems misguided at the very least, and also foolhardy. How can a robot, no matter how well-trained, take the place of a doctor?
Machine learning, the most basic form of artificial intelligence, is already infiltrating the medical field, and it turns out that machines can play an important role in improving our health—including making diagnoses more accurately and quickly and finding better treatments that save people time and money and prevent exposure to harmful side effects. In fact, with modern medicine increasingly dependent on large numbers of studies and drug options and reams of new information, machines may be better able to keep up with and interpret data than the human mind.
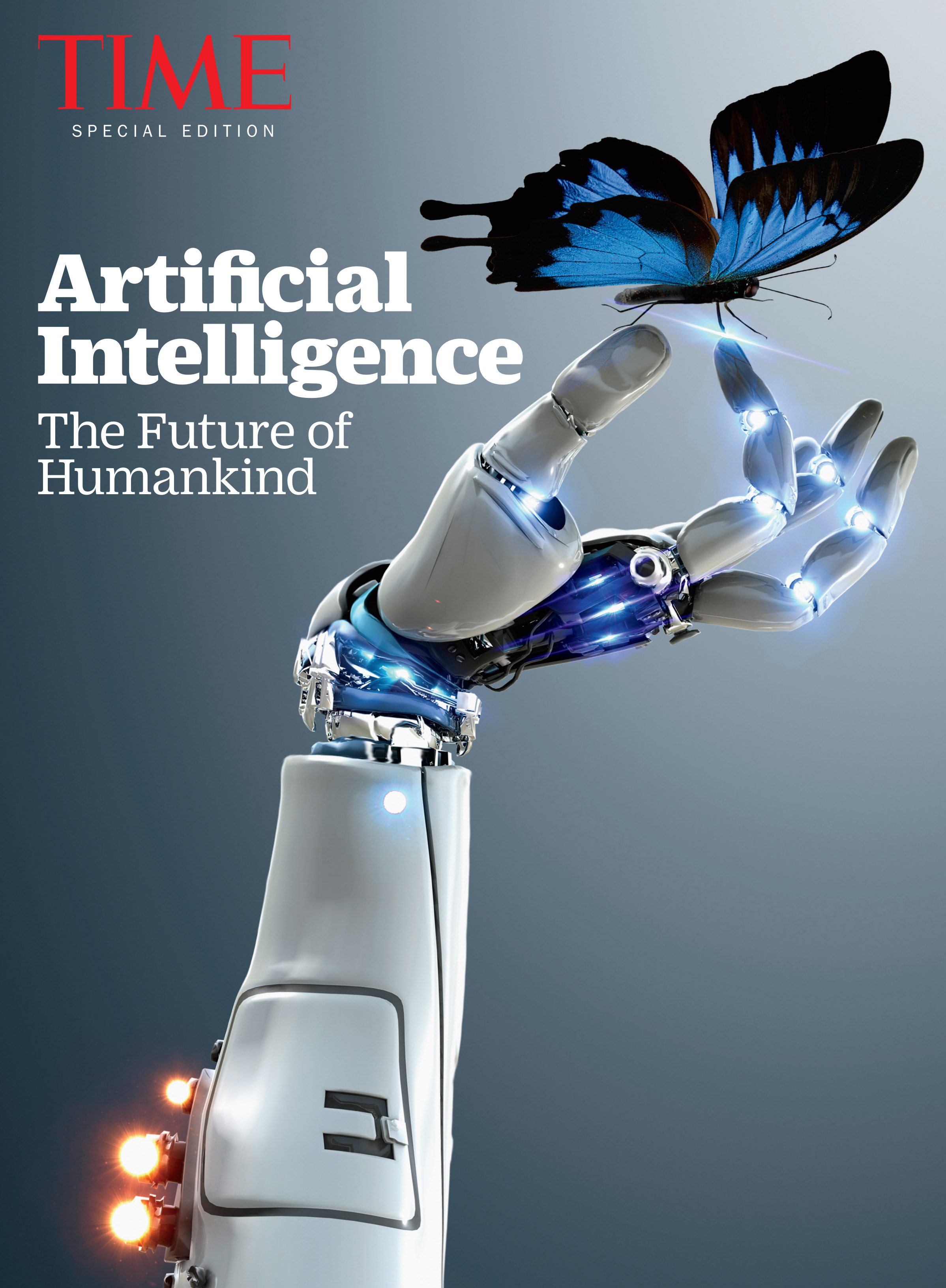
The idea behind artificial intelligence in medicine is not so much to replace the doctor (at least not any time in the near future) but to enhance the doctor’s medical expertise. A.I. programs take the amassed knowledge that every good physician has—which is the product of everything she learned in medical school and in training as well as her experience in treating patient after patient—and scale it to unprecedented levels.
Why should patients have access to just one particular doctor’s expertise when it’s now possible to provide them with the brainpower of hundreds of thousands? Why should patients in rural areas who live geographically far from the nation’s leading medical centers be deprived of all the up-to-date knowledge housed there? “The way artificial intelligence starts to really impact what’s going on in health care is to be able to start cloning all the expert knowledge, so now all of a sudden you get access to all types of care, anywhere,” says Steve Harvey, vice president of Watson Health at IBM.
And with the amount of data available to physicians today—from information about disease symptoms to new drugs, interactions between different drugs and how different people treated in the same way can have very different outcomes—the ability to access and digest information is fast becoming a required skill. And it’s one that machine learning is uniquely designed to master. “Doctors are realizing that if they want to make sense of massive amounts of data, machine learning is a way of allowing them to learn from that data,” says Francesca Dominici, a professor of biostatistics at the Harvard T.H. Chan School of Public Health and co-director of the Data Science Initiative at the university.
Harvard isn’t the only academic institution exploring how man and machine can better combine their skills to exploit unprecedented amounts of medical information. At the University of Texas MD Anderson Cancer Center, the APOLLO program is sifting through the genetic data generated by every patient’s cancer and directing doctors to the treatments that will give their patients the best chance of surviving longer. At the Boston company Neurala, researchers are busy replicating, in silicon, the neural network of the human brain in all its complexity and sophistication. “Today we can design the brain with the complexity of the mouse, which is incredibly smart,” says Massimiliano Versace, Neurala’s CEO. “Science and technology are now aligned for the perfect storm to make artificial intelligence possible.” And in the mental-health field, startups are jumping into machine-learning apps that can help detect when people with conditions such as depression or bipolar disorder are on the verge of a new episode of symptoms in a way that no psychiatrist, however dedicated, ever could.
The key to machine learning in medicine is, well, the machine. And machines from IBM and Google have recently flexed their cognitive muscle by besting the leading Jeopardy! champions, chess masters and Go experts—after learning from the knowledge of previous players, which became part of the machines’ programming.
Now IBM is bringing that idea to medicine, based on the concept that medical knowledge could be as programmable and amenable as the many possible iterations of chess moves and trivia answers. The company is working with experts at Memorial Sloan Kettering Cancer Center in New York to develop IBM Watson for Oncology, made up of three products that address different types of cancer patients. One level will focus on providing patients with the best available information for treating their cancer with existing therapies; Watson provides access to a database of the collected knowledge of Memorial Sloan Kettering’s cancer doctors, as well as the most important cancer studies in the medical literature that these doctors rely on when making their decisions about how to treat patients.
The system incorporates patients’ symptoms and other salient information, such as their family history of the disease and the stage of their cancer, before offering up three different levels of treatment options that the physician can consider. These range from current standard therapies that have already been approved for that type of cancer to treatments approved for other cancers that are currently being tested but are not yet approved for the patient’s specific cancer, and finally truly experimental treatments that some early studies hint might be effective at treating the disease. The different levels of options give both the doctor and patient a treatment plan—if the standard therapies don’t work, then they can move on to the less proven and more experimental ones.
Beyond available treatments, Watson is also helping people with more-advanced cancers who have exhausted the standard therapies. For them, machine learning can call up clinical trials of new therapies that might be effective, including genetic solutions, which are just emerging as a promising area of cancer treatment. The genetic options are based on a careful analysis of the patient’s specific tumor, the mutations driving the disease and drugs that might be targeted to address those mutations.
For human doctors to digest all this information would be nearly impossible, given the demands on physicians’ time to see patients and keep up to date on the latest advances in their field. The potential benefit of having a Watson “doctor” on call at every cancer hospital, no matter how small, can’t be overstated. People with rarer cancers that their local physicians haven’t treated before won’t have to travel great distances to a major hospital that has more experience with that disease, or have to miss the opportunity to get their cancer treated at all. Doctors with less experience with specific cancers can also care for their patients with more confidence, since they now have the institutional knowledge of leading experts in their field at their disposal.
As more information about different cancer patients and their tumors becomes part of Watson, doctors will be able to see patterns that will help them match specific patient profiles to survival rates and better outcomes. They will be able to recognize when people with similar genetic tumors, for example, who took different treatment paths have different health outcomes. That analysis would lead to more refined advice for people about which treatment route is best for them.
The system isn’t perfect yet. Some of IBM’s partners have found Watson cumbersome when it comes to entering all the relevant information from patients—mimicking, in other words, the way doctors incorporate everything they know about a patient into their treatment recommendations. But physicians support the idea that having a way to collect, collate and categorize the massive amounts of information being generated about each patient will be a big part of improving cancer care in coming years.
Such machine-learning approaches are proving remarkably helpful in another area of medicine that may not seem to be so appropriate for non-human interaction: mental health. For people suffering from depression and bipolar disorder, for example, one of psychiatrists and therapists’ most important roles is to help them avoid descending into emotional spirals from which it’s difficult to recover. Identifying when people are most vulnerable to depressive or manic episodes could keep them from the most harmful mental symptoms, and it turns out that machines—in this case a smartphone—might be able to do that better than any psychiatrist can.
That’s because, as is well-known, people verging on depressive episodes or succumbing to feelings of sadness and negativity have changes in their speech and behavior. They may speak less and, when they do, adopt a flat, monotonic tone. They may also disengage from friends and loved ones, calling them less or interacting less frequently on social media. Even the best psychiatrist can’t possibly keep up with all of his patients to monitor when they start to display such changes in behavior. But smartphones can.
Cogito, a mental-health app built on the idea of machine learning, is now being tested at facilities like Brigham and Women’s Hospital in Boston. The app, once installed on a smartphone, monitors activity on social media and phone calls to discern patterns of communication so that when depressive episodes strike, for example, and those patterns change, the app will detect it.
The app also contains a voice analyzer that can search vocal patterns for changes in affect and tone, which may be the first signs of a depressive episode. “The A.I. aspect may be in a better position to gather data over time and give us a better indicator of risk for someone having a mental-health problem and whether they warrant direct intervention with a clinician,” says David Ahern, the director of the Program in Behavioral Informatics and eHealth in the Department of Psychiatry at Brigham.
Machine learning could be especially helpful in alerting a physician or a patient’s family when things begin to spiral out of control. “Historically, we have been dismally poor at detecting dangerousness or self-harm,” says Ahern. “Potentially, with technology like Cogito, we may be able to develop an early-warning system that, for somebody who has a high risk profile because they have a history of depression or suicide attempts, could monitor and see changes in patterns to better determine when the risk gets to the level where intervention is needed to prevent episodes of self-harm or dangerous activity. That’s a place where we haven’t—with traditional models of care—been very good at. We’ve been very reactive, and we want to be more proactive.”
That’s where artificial intelligence can provide the most benefit to people’s health. Its ability to predict how aggressive or mild a person’s disease might be, and to know which treatments might work well and which might not, may make machine learning an integral, and eventually indispensable, part of medical care. It may be time to realize that it’s not man against machine but man and machine together that can finally create the biggest improvements in human health.
More Must-Reads from TIME
- Cybersecurity Experts Are Sounding the Alarm on DOGE
- Meet the 2025 Women of the Year
- The Harsh Truth About Disability Inclusion
- Why Do More Young Adults Have Cancer?
- Colman Domingo Leads With Radical Love
- How to Get Better at Doing Things Alone
- Michelle Zauner Stares Down the Darkness
Contact us at letters@time.com