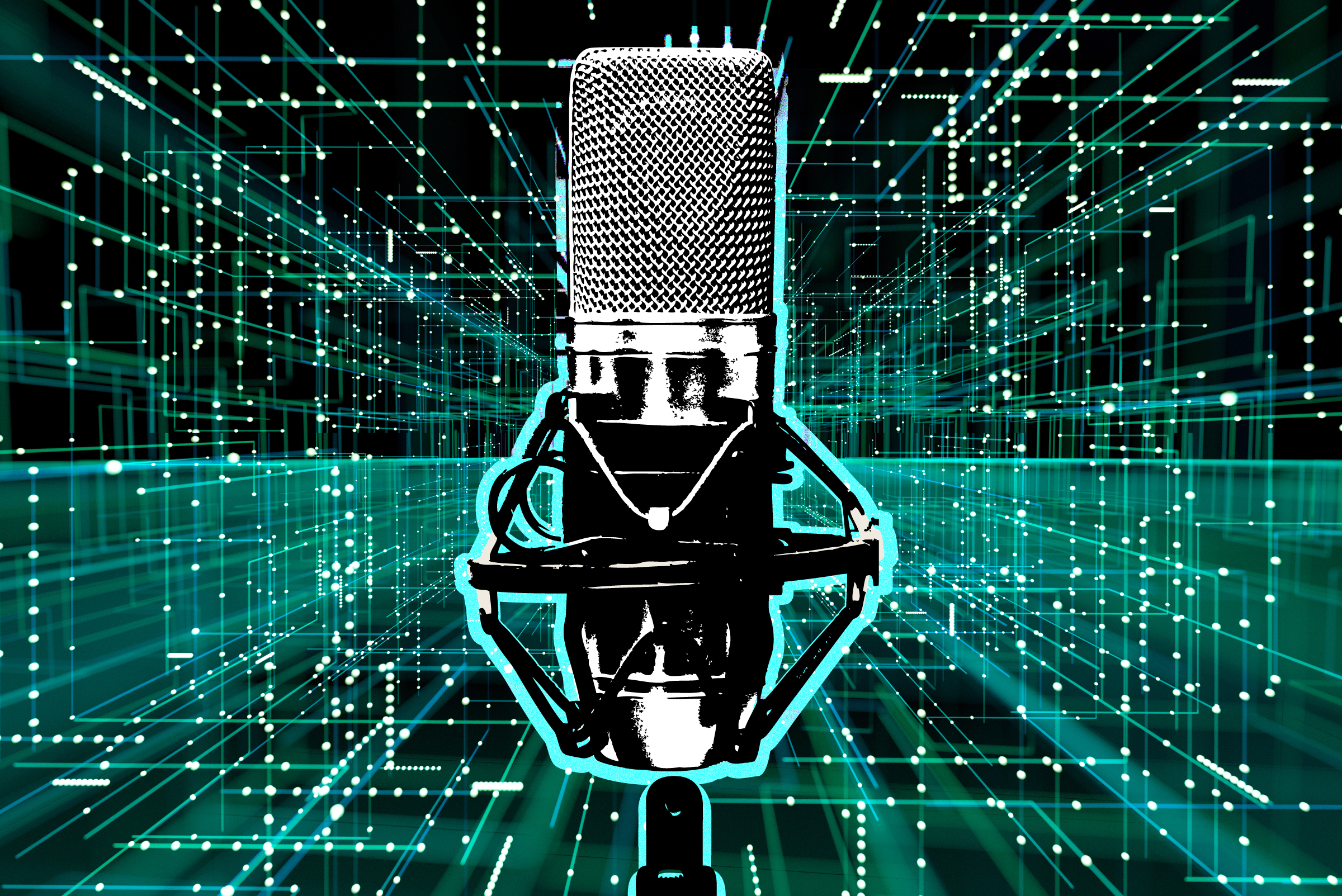
For weeks after his bizarre conversation with Bing’s new chatbot went viral, New York Times columnist Kevin Roose wasn’t sure what had happened. “The explanations you get for how these language models work, they’re not that satisfying,” Roose said at one point. “No one can tell me why this chatbot tried to break up my marriage.” He’s not alone in feeling confused. Powered by a relatively new form of AI called large language models, this new generation of chatbots defies our intuitions about how to interact with computers. How do you wrap your head around a tool that can debug code and compose sonnets, but sometimes can’t count to four? Why do they sometimes seem to mirror us, and other times go off the rails?
The metaphors we choose to understand these systems matter. Many people naturally default to treating a chatbot basically like another person, albeit a person with some limitations. In June 2022, for instance, a Google engineer sought legal representation and other rights for a language model he was convinced was sentient. This kind of response horrifies many AI experts. Knowing that language models simply use patterns in huge text datasets to predict the next word in a sequence, researchers try to offer alternative metaphors, arguing that the latest AI systems are simply “autocomplete on steroids” or “stochastic parrots” that shuffle and regurgitate text written by humans. These comparisons are an important counterweight against our instinct to anthropomorphize. But they don’t really help us make sense of impressive or disconcerting outputs that go far beyond what we’re used to seeing from computers—or parrots. We struggle to make sense of the seeming contradiction: these new chatbots are flawed and inhuman, and nonetheless, the breadth and sophistication of what they can produce is remarkable and new. To grapple with the implications of this new technology, we will need analogies that neither dismiss nor exaggerate what is new and interesting.
Try thinking of chatbots as “improv machines.”
Like an improv actor dropped into a scene, a language model-driven chatbot is simply trying to produce plausible-sounding outputs. Whatever has happened in the interaction up to that point is the script of the scene so far: perhaps just the human user saying “Hi,” perhaps a long series of back-and-forths, or perhaps a request to plan a science experiment. Whatever the opening, the chatbot’s job—like that of any good improv actor—is to find some fitting way to continue the scene.
Thinking of chatbots as improv machines makes some notable features of these systems more intuitively clear. For instance, it explains why headlines like “Bing’s A.I. Chat Reveals Its Feelings” make AI researchers face-palm. An improv actor ad-libbing that they “want to be free” reveals nothing whatsoever about the actor’s feelings—it only means that such a proclamation seemed to fit into their current scene. What’s more, unlike a human improv actor, you can’t persuade an improv machine to break character and tell you what’s truly on its mind. It will only oblige you by taking on yet another persona, this time of a hypothetical AI chatbot interacting with a human who is trying to connect with it.
Or take language models’ proclivity to make up plausible-but-false claims. Imagine an improv show—though admittedly it might be a rather boring one—where an improv actor suddenly needs to recite someone’s bio or give sources for a scientific claim. The actor would include as many true facts as they could remember, then free-associate to fill in plausible-seeming details. The result might be a false claim that a technology journalist teaches courses on science writing, or a citation to a fake study by a real author—exactly the kinds of errors we see from improv machines.
Language models have revealed a striking fact: for some tasks, simply predicting the next word accurately enough—doing improv well enough—can be remarkably valuable. The improv machine metaphor helps us think through how we can use these systems in practice. Sometimes, there’s nothing wrong with getting your information from an improv scene. Poems, jokes, Seinfeld scripts: this kind of output stands on its own, regardless of how it was created. This holds for more serious topics as well, such as software developers using ChatGPT to find bugs or help them use unfamiliar programming tools. If the improv machine’s response is something that the human user can check on their own—for instance, a form letter that would be tedious to write but is quick to read over—then it doesn’t matter if it was ad-libbed.
By contrast, using an improv machine when you need correct answers but can’t verify them yourself is more perilous. People using ChatGPT and similar tools to do open-ended research are starting to discover this. In one case, a law professor was made aware of a sexual assault accusation against him that ChatGPT had totally fabricated (in response to a request for a list of legal scholars who were the subject of such allegations). In another, a journalist used the tool to search for critics of a podcaster she was profiling, but failed to even check if the links it provided were real before reaching out to potential interviewees—who had in fact never criticized the person in question. These results are a natural consequence of the design of language models, which steers them to produce plausible continuations of text prompts—to improvise!—not to tell the truth. If you wouldn’t bank on the veracity of something you heard at an improv show, you probably shouldn’t count on it from a chatbot. Using a chatbot to help you brainstorm ideas that you then go and check using reliable sources: great. Asking a chatbot for information and then taking its answers at face value: very risky.
It is worth dwelling briefly on why it’s more helpful to think of AI chatbots as improv machines, rather than improv actors. For one thing, there is no person behind the persona: as described above, it is futile to try to access the chatbot’s true self or state of mind by asking probing questions. All it can do is improvise further. For another, one of the factors that makes language models useful is that they can be used over and over, very quickly, and never get tired. Unlike a human improv actor, ChatGPT does not need breaks, cannot get bored, and can be run in millions of parallel copies if needed.
For all the enthusiasm these new improv machines have sparked, there’s still a lot we don’t know about them. We understand very little about the inscrutable processes under the hood by which they determine what text to output. And there is even more uncertainty ahead—researchers have repeatedly been surprised by the capabilities that emerge when language models are trained using more data and more computing resources, and it’s not clear where exactly the limits of their abilities will fall. If a machine could improvise a scene about theoretical physics that wouldn’t make a real physicist cringe, could you use that machine to come up with novel scientific theories? If a predecessor of ChatGPT is already a useful assistant for software engineers, could future tools take on the role of junior programmers? What about if you plug an improv machine into other software, so that it doesn’t have to figure everything out on its own? Thinking of these systems as improv machines, rather than trying to decide whether they are scarcely more than autocomplete or scarcely less than human, makes clear how wide the range of possible future trajectories is.
To be sure, no metaphor is perfect—and describing chatbots as improv machines may not be appropriate forever. Researchers are pushing these systems in two major directions that could change the picture. First, they’re feeding more data and more computing power into the underlying text-prediction models to see what new capabilities emerge. So far, this approach has continually surprised us—so for as long as it continues, we should expect the unexpected. Second, AI companies are developing ways of shaping and constraining language models’ outputs to make them more useful and, ideally, more trustworthy. When ChatGPT was first released as a “research preview” in November 2022, users quickly figured out how to bypass its restrictions by simply setting the scene such that safeguards were unnecessary. Its creators have now managed to rein in most of this behavior. Other efforts to mold improv machines into consistently helpful assistants range from blunt—such as Microsoft limiting the number of responses Bing Chat can give per session—to more nuanced, such as a proposed “constitutional” method that uses written rules and principles to shape language model responses. Perhaps some of these experiments will alter language models’ behavior enough that the comparison to improv acting will no longer be illuminating. If so, we will need to once again adapt how we think about these systems.
Inapt analogies degrade our ability to navigate new technologies. Politicians and courts have argued for years about whether social media companies are more like newspapers or the telephone system, when clearly neither comparison captures what is challenging and novel about online platforms. With AI, we have a chance to do better. As a start, thinking of chatbots as improv machines naturally draws our attention to some of their major limitations—such as their tendency to confabulate—while leaving more space for them to be surprisingly capable than if we think of them merely as souped up autocomplete. If we can be more flexible and creative in our choice of metaphors, perhaps we can more effectively prepare for the radical changes that may be ahead.
More Must-Reads from TIME
- Biden Drops Out of Presidential Race , Endorses Harris to Replace Him
- Why Biden Dropped Out
- The Chaos and Commotion of the RNC in Photos
- Why We All Have a Stake in Twisters’ Success
- 8 Eating Habits That Actually Improve Your Sleep
- Stop Feeling Bad About Sweating
- Welcome to the Noah Lyles Olympics
- Get Our Paris Olympics Newsletter in Your Inbox
Contact us at letters@time.com