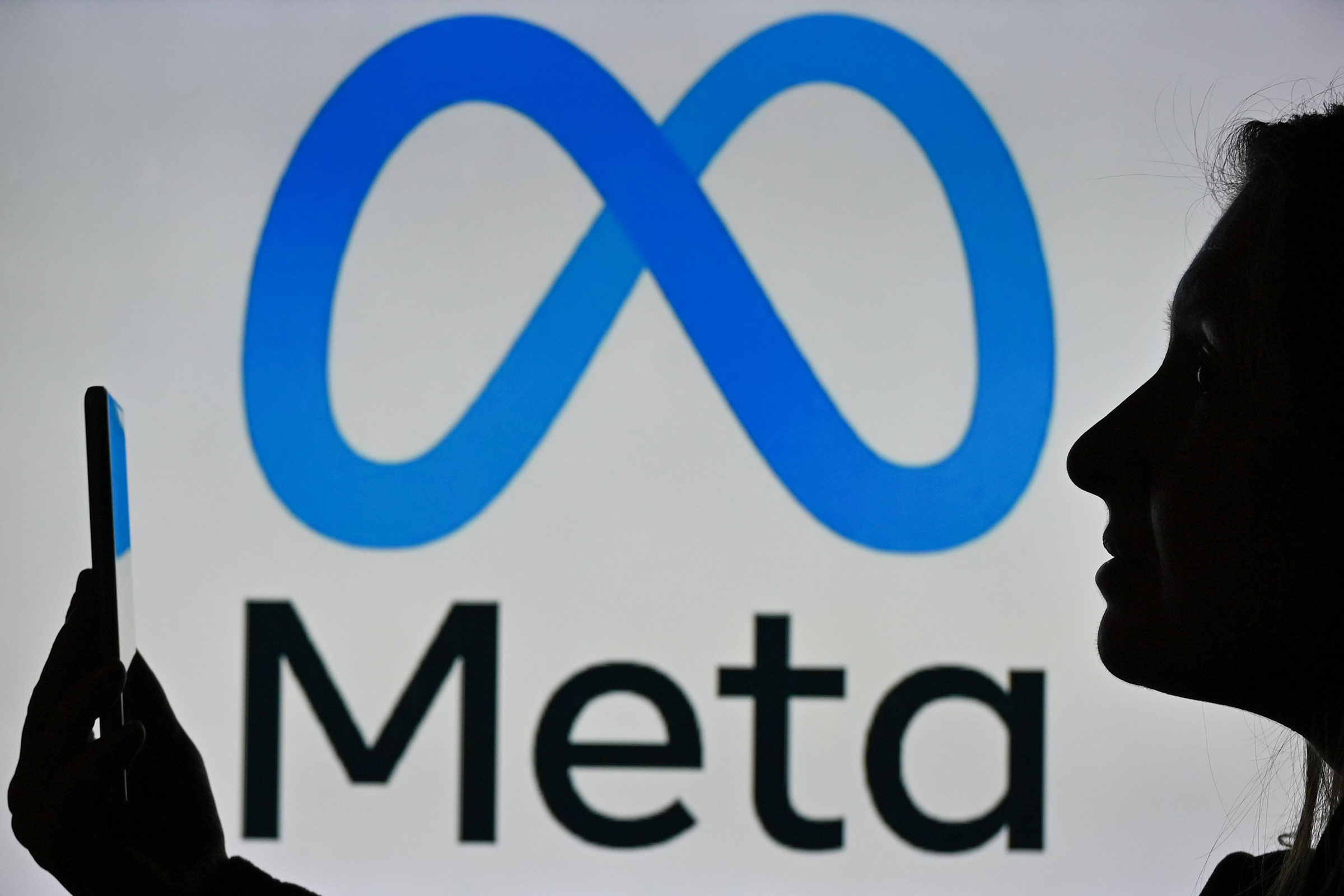
Researchers at Meta, the parent company of Facebook, are working on a new way to understand what’s happening in people’s minds. On August 31, the company announced that research scientists in its AI lab have developed AI that can “hear” what someone’s hearing, by studying their brainwaves.
While the research is still in very early stages, it’s intended to be a building block for tech that could help people with traumatic brain injuries who can’t communicate by talking or typing. Most importantly, researchers are trying to record this brain activity without probing the brain with electrodes, which requires surgery.
The Meta AI study looked at 169 healthy adult participants who heard stories and sentences read aloud, as scientists recorded their brain activity with various devices (think: electrodes stuck on participants’ heads).
Researchers then fed that data into an AI model, hoping to find patterns. They wanted the algorithm to “hear” or determine what participants were listening to, based on the electrical and magnetic activity in their brains.
TIME spoke with Jean Remi King, a research scientist at Facebook Artificial Intelligence Research (FAIR) Lab, about the goals, challenges, and ethical implications of the study. The research has not yet been peer-reviewed.
This interview has been condensed and edited for clarity.
TIME: In layman’s terms, can you explain what your team set out to do with this research and what was accomplished?
Jean Remi King: There are a bunch of conditions, from traumatic brain injury to anoxia [an oxygen deficiency], that basically make people unable to communicate. And one of the paths that has been identified for these patients over the past couple of decades is brain-computer interfaces. By putting an electrode on the motor areas of a patient’s brain, we can decode activity and help the patient communicate with the rest of the world…But it’s obviously extremely invasive to put an electrode inside someone’s brain. So we wanted to try using noninvasive recordings of brain activity. And the goal was to build an AI system that can decode brain responses to spoken stories.
What were the biggest challenges you came up against in the process of conducting this research?
There are two challenges I think worth mentioning. On the one hand, the signals that we pick up from brain activity are extremely “noisy.” The sensors are pretty far away from the brain. There is a skull, there is skin, which can corrupt the signal that we can pick up. So picking them up with a sensor requires super advanced technology.
The other big problem is more conceptual in that we actually don’t know how the brain represents language to a large extent. So even if we had a very clear signal, without machine learning, it would be very difficult to say, “OK, this brain activity means this word, or this phoneme, or an intent to act, or whatever.”
So the goal here is to delegate these two challenges to an AI system by learning to align representations of speech and representations of brain activity in response to speech.
What are the next steps to further this research? How far away are we from this AI helping people who have suffered a traumatic neurological injury communicate?
What patients need down the line is a device that works at bedside and works for language production. In our case, we only study speech perception. So I think one possible next step is to try to decode what people attend to in terms of speech—to try to see whether they can track what different people are telling them. But more importantly, ideally we would have the ability to decode what they want to communicate. This will be extremely challenging, because when we ask a healthy volunteer to do this, it creates a lot of facial movements that these sensors pick up very easily. To be sure that we are decoding brain activity as opposed to muscle activity will be very difficult. So that’s the goal, but we already know that it’s going to be very hard.
How else could this research be used?
It’s difficult to judge that because we have one objective here. The objective is to try to decode what people have heard in the scanner, given their brain activity. At this stage, colleagues and reviewers are mainly asking, “How is this useful? Because decoding something that we know people heard is not bringing much to [the table].” But I take this more as a proof of principle that there may be pretty rich representations in these signals—more than perhaps we would have thought.
Is there anything else you think it’s important for people to know about this study?
What I would like to stress is that this is research that is performed within FAIR and, in that regard, is not directed top-down by Meta and is not designed for products.
More Must-Reads From TIME
- The 100 Most Influential People of 2024
- Coco Gauff Is Playing for Herself Now
- Scenes From Pro-Palestinian Encampments Across U.S. Universities
- 6 Compliments That Land Every Time
- If You're Dating Right Now , You're Brave: Column
- The AI That Could Heal a Divided Internet
- Fallout Is a Brilliant Model for the Future of Video Game Adaptations
- Want Weekly Recs on What to Watch, Read, and More? Sign Up for Worth Your Time
Write to Megan McCluskey at megan.mccluskey@time.com