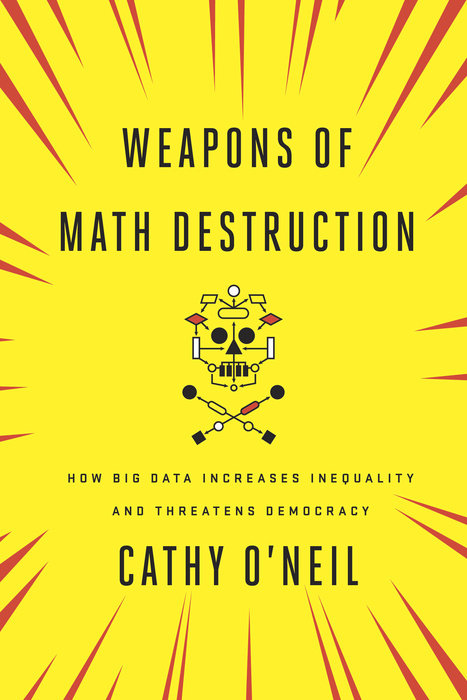
Ever wonder why your children’s teachers are so focused on their test scores, at times seemingly to the exclusion of all else? There are lots of reasons, from the educational reform movement to a greater emphasis on quantitative metrics in American schools, but one of the least explored and most important reasons is that test scores are very often fed into algorithms that schools then use to judge teachers themselves. These “value-added” models, which use children’s scores to gauge how well their instructors are doing, try to account for the variance in performance between rich and poor neighborhoods by measuring not just absolute scores, but more nebulous estimates of where kids are, and where they could be. A laudable goal, but such models also depend on shallow and volatile data sets that attempt to map how kids “should” be performing. It’s no surprise, given all the subjective inputs, that teacher scores can vary wildly from year to year. And yet, these models are a crucial part of hiring and firing decisions in many parts of the country.
In fact, these are the very same kind of algorithmic models that were used during the subprime crisis to generate disastrously flawed ideas about which mortgage holders were likely to default, and which weren’t. The point here is that while we increasingly look to Big Data to tell us who should be hired and fired, or be given credit, or a job, or delivered the latest advertisement for luxury goods versus discount products, the mathematical models making these choices are hardly infallible.
Algorithms don’t necessarily lead us to truth; in fact, they are quite subjective, as a spate of new examples of race and class bias via computer modeling has shown. Last year, Amazon came under fire for algorithmic models that limited its Prime delivery service in many minority neighborhoods (the company has since tried to rectify the issue). In both the U.S. and the U.K., financial institutions have been penalized for computer-generated decisions that discriminate against credit or insurance seekers by race. New Jersey-based Hudson City Savings Bank, for example, was recently fined $33 million for using zip code data to redline borrowers.
The problem has been building for some time. Back in May 2014, the White House released a report titled Big Data: Seizing Opportunities, Preserving Values, which found that “big data analytics have the potential to eclipse longstanding civil rights protections in how personal information is used in housing, credit, employment, health, education, and the marketplace.” But the effects are hard to track, because like the dark financial arts employed in the run up to the 2008 subprime housing crisis, the Big Data algorithms that sort us into piles of “worthy” and “unworthy” are mostly opaque and unregulated, not to mention generated (and used) by large multinational firms with huge lobbying power to keep it that way. “The discriminatory and even predatory way in which algorithms are being used in everything from our school system to the criminal justice system is really a silent financial crisis,” says Cathy O’Neil, the author of Weapons of Math Destruction, a new book that tracks the effects of computerized discrimination across a variety of sectors of society and the economy.
Her work makes particularly disturbing points about how being on the wrong side of an algorithmic decision can snowball in incredibly destructive ways—a young black man, for example, who lives in an area targeted by crime-fighting algorithms that add more police to his neighborhood because of higher violent crime rates, will be more likely to be targeted for any petty violation, which adds to a digital profile that could subsequently limit his credit, his job prospects, and so on. Yet neighborhoods more likely to commit white-collar crime aren’t targeted in this way. In higher education, the use of algorithmic models that rank colleges has led to an educational arms race where schools offer more and more merit rather than need-based aid to students who’ll make their numbers (and thus rankings) look better.
So far, the big and small tech companies that create the software, not to mention the myriad firms that use it, have been reluctant to get out in front of the issue. “Companies are both complacent and terrified, and hoping it will all just go away,” says Trevor Phillips, the former head of the U.K.’s Equality and Human Rights Commission, who now runs an analytics firm dedicated to using big data to expand diversity. It won’t. If you haven’t yet been on the wrong side of Big Data, the numbers make it likely that you will be someday.
How do we fix things? O’Neil, a former quant trader turned social activist, has proposed a Socratic Oath for mathematicians. Others suggest random algorithmic “audits” by regulators or rules that would make “must have” services like credit, housing, professional licenses and so on “data blind” when it comes to race or gender. One thing is already very clear—far from being coolly scientific, Big Data comes with all the biases of its creators.
More Must-Reads from TIME
- Why Biden Dropped Out
- Ukraine’s Plan to Survive Trump
- The Rise of a New Kind of Parenting Guru
- The Chaos and Commotion of the RNC in Photos
- Why We All Have a Stake in Twisters’ Success
- 8 Eating Habits That Actually Improve Your Sleep
- Welcome to the Noah Lyles Olympics
- Get Our Paris Olympics Newsletter in Your Inbox
Contact us at letters@time.com