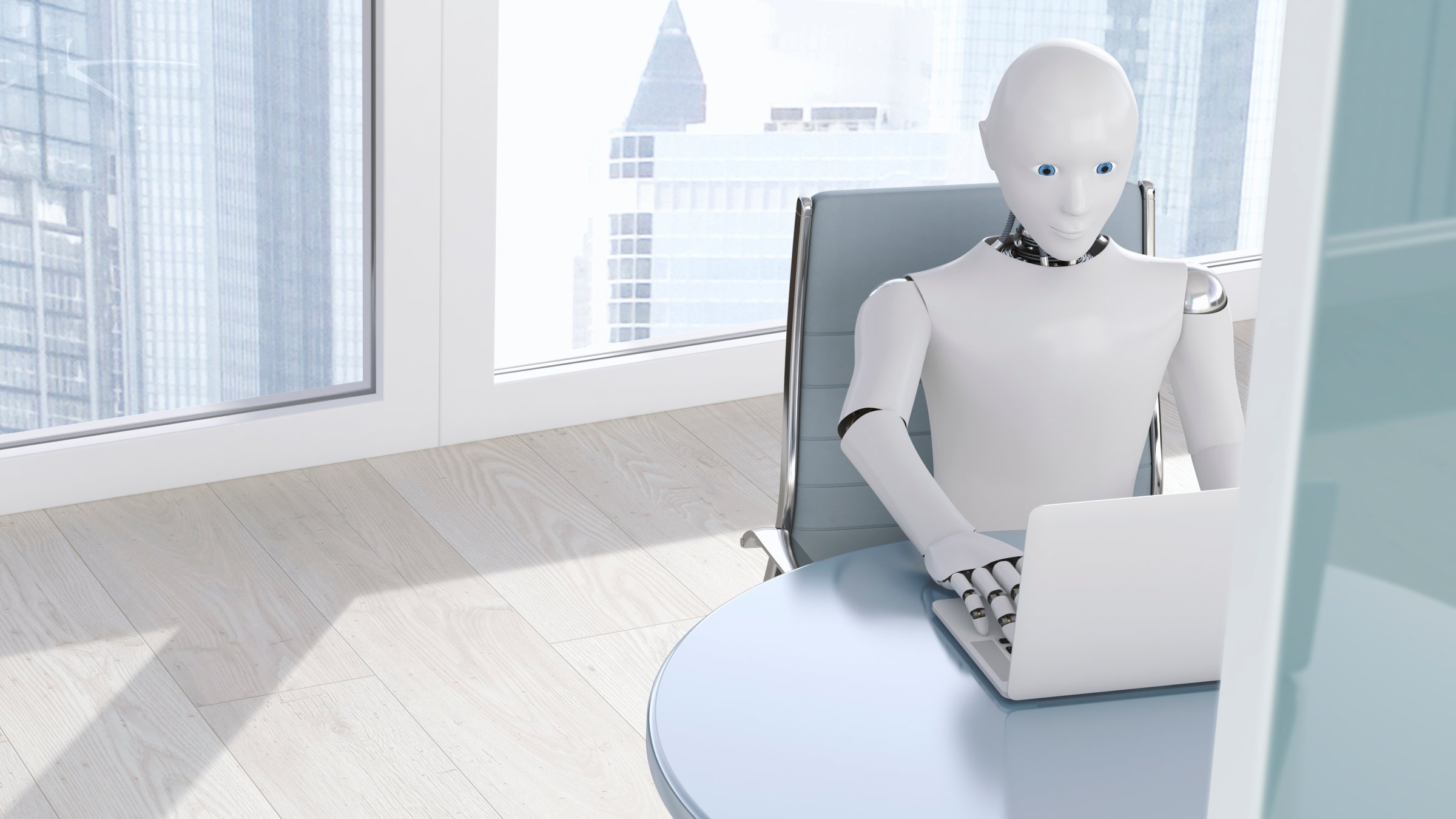
There’s no shortage of research showing that women and people of color get worse treatment than their white male peers in the job market. In one well-known study, academic institutions across the country rated a résumé as more qualified for a lab manager position — and suggested a higher starting salary — when the name at the top read “John” instead of “Jennifer.” Just last month, job platform Hired compared salary data across tech-industry workers and found a similar result: 63% of the time, their study reported, women were offered a lower starting salary than men for the same position at the same company. And a new meta-analysis of two dozen studies related to race and hiring performed since 1989 showed that equally qualified black job candidates get 36% fewer callbacks than their white counterparts. Even more horrifying, this statistic hasn’t seen any meaningful change in 25 years.
Now, a whole host of tech companies are cropping up promising to remove these types of biases from hiring — with the help of artificial intelligence. There’s Koru, which uses surveys to identify current employees’ strengths and weaknesses, and then looks for those same traits in applicants. There’s Pymetrics, which uses “gamified neuroscience and A.I.” to predict success, and then find applicants who fit the same profile. And there’s Ideal, which uses AI to screen résumés and cherry-pick candidates. All these products promise to help companies diversify or eliminate bias — and more are coming.
AI-enabled hiring software may be a booming market, but I won’t be trusting it to level the playing field or eliminate the wage gap anytime soon. Because for all their seemingly scientific methods, algorithms aren’t neutral at all. They’re just as fallible as the humans who made them — and they can easily reinforce all those biases we say we’re trying to get rid of. In fact, if you train AI to be biased, it can actually get worse over time, not better — optimizing for those same biases over and over.
The concept of algorithmic bias affecting employment isn’t new, either. Back in the summer of 2015, researchers from Carnegie Mellon and the International Computer Science Institute wanted to learn more about how Google’s ad-targeting algorithms worked. So they built a piece of software called AdFisher, which simulates web-browsing activities, and set it to work gathering data about the ads shown to fake users with a range of profiles and browsing behaviors. The results were startling: the profiles Google had pegged as male were much more likely to be shown ads for high-paying executive jobs than those Google had identified as female — even though the simulated users were otherwise equivalent.
So how can we ensure AI is a boon for marginalized groups, rather than just a shiny new way to reify the same old problems? It all depends on what, exactly, the AI does — and how it learned to do it.
For example, consider resume-screening tools. This type of software relies on natural-language processing — that is, a computer’s ability to understand human language as it’s actually spoken or written. To get language right, though, machines need a lot more than a dictionary. They need to understand all the nuance that goes into human communication.
That’s where tools like Word2vec come in. This technology, which was built by Google researchers, looks at the relationships, or vectors (that’s the “vec” in its name), between words. For example, which words tend to appear in the same texts? How far from each other do two words tend to appear? From there, it learned about the semantic relationships between those words and can correctly complete all kinds of analogies, like “Paris is to France as _____ is to Japan.” But the system also returns some less factual — and deeply frustrating — answers, like, “Man is to computer programmer as woman is to homemaker.” Why? Because Word2vec learned about words by being fed a huge number of Google News articles. So when the algorithm crunched through all that historical data about people and culture, it didn’t come out with just the facts. It came out with a set of relationships deeply influenced by historical biases and norms.
The problem isn’t that tools like Word2vec exist, or that they reflect the data they were given. The problem is that so many of us take their results at face value: the results can’t be biased — the machine calculated them! But say you fed the algorithm nothing but help-wanted ads from the 1950s: would you want that picture of society — one where ads explicitly excluded black folks, or dictated what a woman applicant should look like — to be what a machine tries to replicate? Of course not. The biases in contemporary Google News articles might be less overt, but they’re certainly not neutral. They reflect the stories and people that someone deemed important enough to cover — and can even include damaging sources like racist websites and fake news.
In fact, even software designed to look at the personality traits of your best employees and hire more of the same has that problem — after all, if your past employment practices were biased, your understanding of who’s a good fit is necessarily limited. If all the personalities a company considers “ideal” just so happen to belong to men, how can it know what benefits hiring women might bring?
Meanwhile, most Americans don’t even realize these kinds of algorithmic hiring processes exist. According to a Pew Research report released this month, less than half of Americans have heard anything about this kind of software — and only 12% thought it was “extremely realistic.”
That’s a problem. After all, what worried the researchers in the AdFisher experiment wasn’t just the biased results. It was that they couldn’t figure out exactly why those results happened. That’s because, like most of the systems that power modern technology, Google’s ad-placement algorithms are proprietary: a black box we can bang on, but never quite see inside of. Without transparency and public education, all these so-called bias-busting HR tools rely on blind faith — a trust that the companies behind them have not only good intentions for increasing equality, but also the ability to actually do so.
That’s not a trust I’m willing to extend right now — not to a tech industry infamous for its terrible treatment of women and minorities. And if you’re concerned about fairness at work, neither should you.
Sara Wachter-Boettcher is the author of Technically Wrong: Sexist Apps, Biased Algorithms, and Other Threats of Toxic Tech.
More Must-Reads from TIME
- Welcome to the Noah Lyles Olympics
- Melinda French Gates Is Going It Alone
- What to Do if You Can’t Afford Your Medications
- How to Buy Groceries Without Breaking the Bank
- Sienna Miller Is the Reason to Watch Horizon
- Why So Many Bitcoin Mining Companies Are Pivoting to AI
- The 15 Best Movies to Watch on a Plane
- Want Weekly Recs on What to Watch, Read, and More? Sign Up for Worth Your Time
Contact us at letters@time.com